At the HCI Games Group, we love looking at emotion as a core driver of gameplay experience. One common technique used to find out how players experience a game prototype and what affective responses in-game interaction triggers, is to ask players how they feel after playing the game. For this purpose, different affective dimensions like arousal (i.e., the level of excitement), valence (i.e., good or bad) or dominance (i.e., how much the player felt in control) are often used to quantify subjective phenomena. As you can imagine, these types of self-reports are extremely valuable to iteratively improve a game prototype.
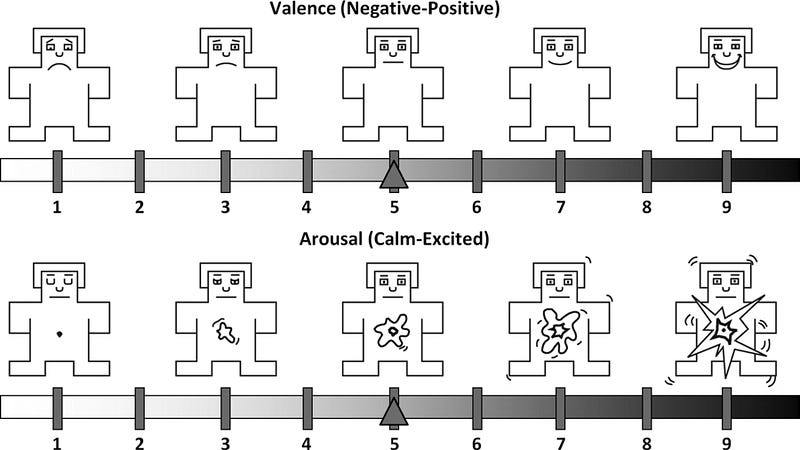
However, one drawback of post-hoc questionnaires is that certain types of emotions are temporary bursts of experience which may fade over time. This becomes a problem if the goal is to investigate affective responses in real-time. To work around this problem, the use of biosignals like brain activity (e.g., through electroencephalography or magnetoencephalography), heart rate (e.g., through electrocardiography), skin conductance level, skin temperature or muscular activity (via electromyograms) has been suggested in the literature [1]. A major focus of the HCI Games Group is in this area.
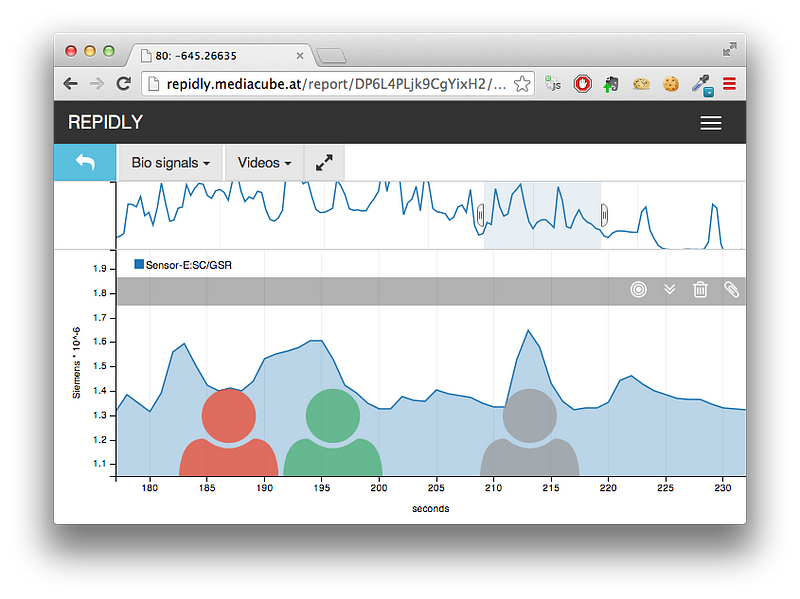
The main challenge of this biometric approach lies in finding a reliable mapping from physiological patterns to affective states as experienced by the player. The goal is to quantify certain affective dimensions in real-time, without interrupting the game flow to interview the player about their emotional state. Therefore, it is helpful to create automated methods to estimate amplitudes of affective dimensions based on real-time physiological measurements on the fly. However, datasets containing all the information needed to create such automated methods, are costly, hard to find online, and tedious to acquire yourself.
In this blog post, we hope to provide a usable synopsis of freely available datasets that can be used by anyone who is interested in doing research in this area. The four datasets we summarize in this post are MAHNOB-HCI (Soleymani et al.), EMDB (Carvalho et al.), DEAP (Koelstra et al.), and DECAF (Abadi et al.). All these datasets were acquired by presenting multimedia content (i.e., images or videos) to the participants, and recording various physiological responses the participants had to this content. To allow for a mapping from physiological to affective responses, all of the datasets contain subjective self-reports about affective dimensions like arousal, valence, and dominance.